Diffusion Models
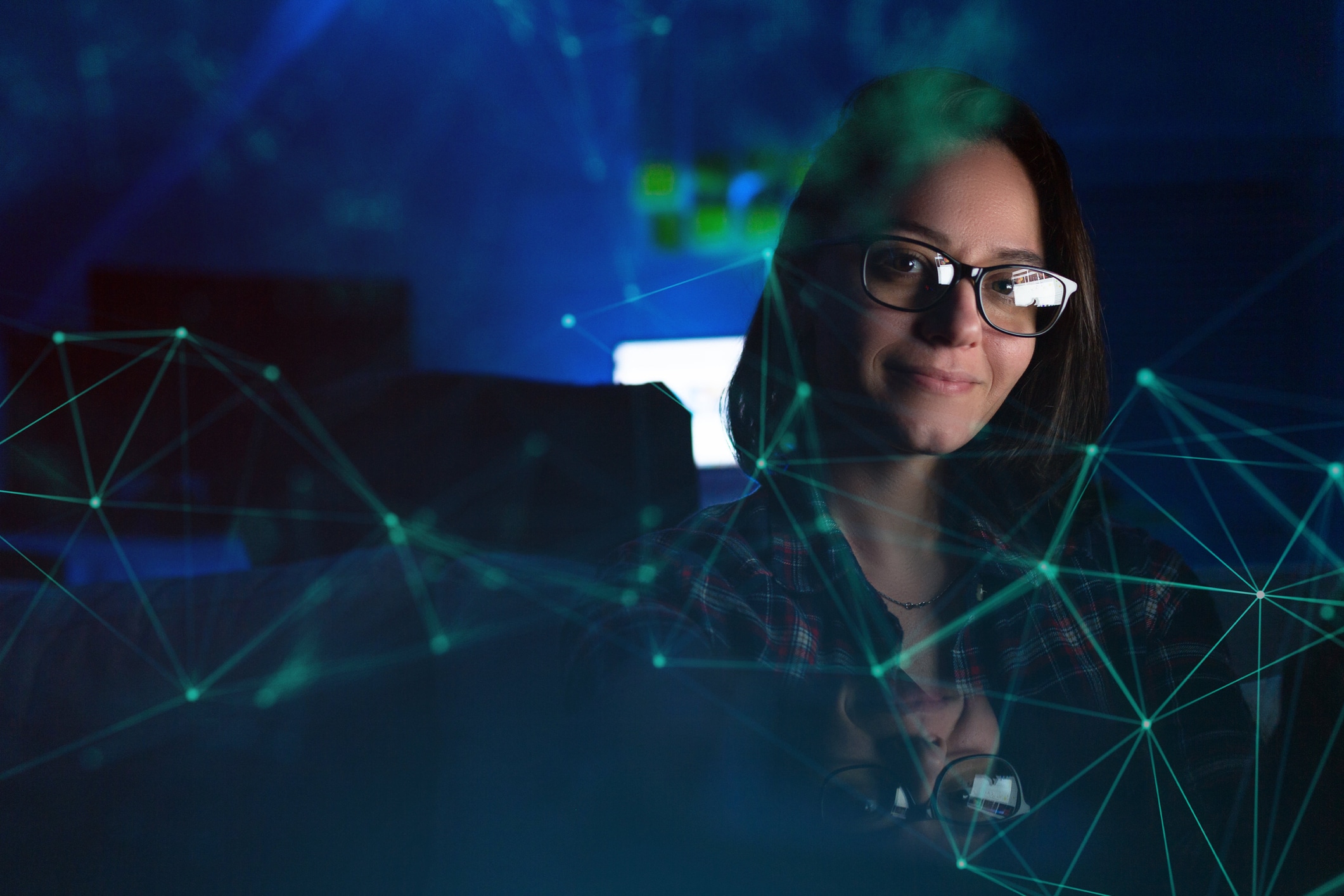
Diffusion models enable Machine Learning (ML) models to create and enhance images and videos. Text-based prompts drive image creation to provide information about the required frame, subject, and style.
Diffusion models work by learning from training datasets and then discarding them after training. They also add noise to an image in a reversible manner, learn to de-noise the image and apply what the model learned to create entirely new images. Generative Pre-trained Transformer (GPT) image tools such as Dall-E2 and Microsoft Designer use diffusion models.
Why are Diffusion Models Important?
Diffusion models have provided an innovative and effective approach to image creation that is considered superior to alternative approaches for creating high-quality images, including generative adversarial networks (GANs), Variational Autoencoders (VAEs), and Flow-based models. Unlike GANs, diffusion models smooth out distributions, resulting in diffusion models having more diversity in images. This means the diffusion model can provide multiple variations of an image compared with the older approaches to image generation and noise reduction. Diffusion models are in their infancy yet are already demonstrating their superiority to traditional approaches.
Developing and Refining Prompts
The frame component of the prompt specifies the required style of the required output. Examples of frames include a drawing, photograph, or oil painting.
The frame is combined with a subject that can be something with lots of internet images available to learn from. For example, if you are in hospitality, you might choose your hotel properties as the subject because your goal is to create abstract imagery for promotions and brochures.
The specified frame and subject can have a style, which might be specified as an art or lighting style of moody, sunny, surrealist, or abstract.
Customizing Images
The generated images can have cutouts to allow additional content placement. Inpainting can replace elements in the image, such as selecting a clothing style, clouds in the sky, or how a person is posed.
Outpainting refers to the ability to create a context for the subject being generated. For example, you may want to place the subject in a certain room or a park setting.
Applications of Diffusion Models
The applications of diffusion models will become increasingly commonplace thanks to products from companies such as Microsoft and OpenAI that are embedding the models in their platforms. Here are use cases that diffusion models enable:
- Diffusion models will transform product design by enabling designers to view designs from multiple angles, apply perspectives, and create 3D renders that can be used to print 3D models.
- Marketers can use text to describe what images they would like to associate with content and have them rendered rather than pay for a compromise stock photo, as is typically done today.
- Online retailers can show products in different settings and different colors.
- Using diffusion model-driven renders, online configurators can create high-resolution images of products such as cars that include custom features and view them in varying settings.
Challenges With Diffusion Models
Diffusion models are still new and evolving quickly. Limitations include:
- Faces can be distorted when more than two people are in an image.
- Text in an image can be distorted.
- Diffusion models perform best when the output is like their training data.
- Diffusion models require massive server resources that can become expensive in cloud environments with metered central processing unit (CPU), graphics processing unit (GPU), and tensor processing unit (TPU) usage. Products such as DreamStudio from Stability AI are open-sourced with a downloadable version that can be run using in-house hardware to avoid metered usage costs.
- Image generation is complex, making the process hard to optimize without the use of lots of additional tagged training data. Often, prompts are misinterpreted, leading to unexpected results.
- AI-based generation is susceptible to bias, just as human trainers are. Care must be taken to constrain models to function within acceptable social and ethical standards.
Try the Actian Data Platform
The Actian Data Platform provides a unified experience for ingesting, transforming, analyzing, and storing data. The Actian Data Platform spans multiple public clouds and can be self-managed on-premises. The built-in data integration technology allows customers to load their data easily to get trusted insights quickly.
The Actian Data Platform provides ultra-fast query performance, even for complex workloads, without tuning. This is due to a highly scalable architecture that uses columnar storage with vector processing for unmatched parallelism for query processing.