Data Preparation Guide: 6 Steps to Deliver High Quality GenAI Models
Dee Radh
March 11, 2024
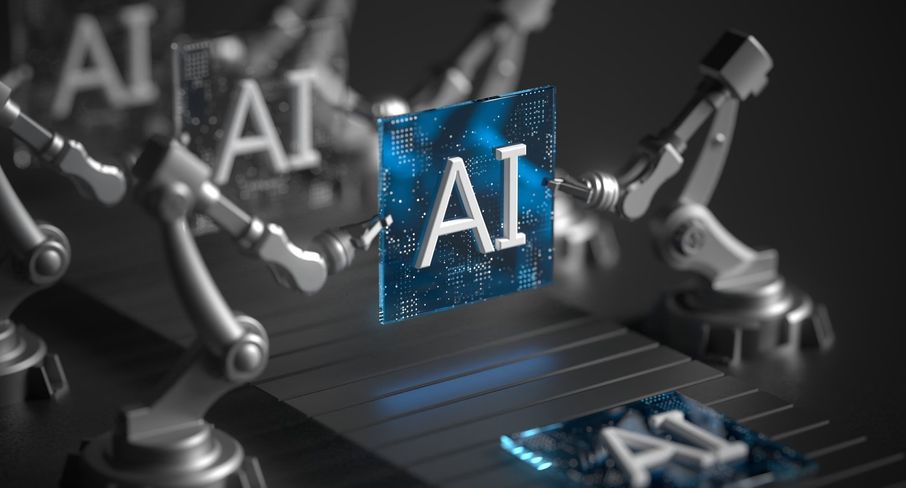
Data preparation is a critical step in the data analysis workflow and is essential for ensuring the accuracy, reliability, and usability of data for downstream tasks. But as companies continue to struggle with data access and accuracy, and as data volumes multiply, the challenges of data silos and trust become more pronounced.
According to Ventana Research, data teams spend a whopping 69% of their time on data preparation tasks. Data preparation might be the least enjoyable part of their job, but the quality and cleanliness of data directly impacts analytics, insights, and decision-making. This also holds true for Generative AI. The quality of your training data impacts the performance of GenAI models for your business.
High-Quality Data Preparation: The Foundation for Successful AI
Generative AI models, such as Generative Adversarial Networks (GANs) or Variational Autoencoders (VAEs), learn from patterns and structures present in the input data to generate new content. To train models effectively, data must be curated, transformed, and organized into a structured format, free from missing values, missing fields, duplicates, inconsistent formatting, outliers, and biases.
Without a doubt, data preparation tasks are a time-consuming and repetitive process. But, failure to adequately prepare data can result in suboptimal performance, biased outcomes, and ethical, legal, and practical challenges for Generative AI applications.
Generative AI models lacking sufficient data preparation may face several challenges and limitations. Here are three major consequences:
Poor Quality Outputs
Generative AI models often require data to be represented in a specific format or encoding in a way that’s suitable for the modeling task. Without proper data preparation, the input data may contain noise, errors, or biases that negatively impact the training process. As a result, Generative AI models may produce outputs that are of poor quality, lack realism, or contain artifacts and distortions.
Biased Outputs
Imbalanced datasets in which certain classes or categories are underrepresented, can lead to biased models and poor generalization performance. Data preparation ensures that the training data is free from noise, errors, and biases, which can adversely affect the model’s ability to learn and generate realistic outputs.
Compromised Ethics and Privacy
Generative AI models trained on sensitive or personal data must adhere to strict privacy and ethical guidelines. Data preparation involves anonymizing or de-identifying sensitive information to protect individuals’ privacy and comply with regulatory requirements, such as GDPR or HIPAA.
By following a systematic checklist for data preparation, data scientists can improve model performance, reduce bias, and accelerate the development of Generative AI applications. Here are six steps to follow:
-
Project Goals
-
- Clearly outline the objectives and desired outcomes of the Generative AI model so you can identify the types of data needed to train the model.
- Understand how the model will be utilized in the business context.
-
Data Collection
-
- Determine and gather all potential sources of data relevant to the project.
- Consider structured and unstructured data from internal and external sources.
- Ensure data collection methods comply with relevant regulations and privacy policies (e.g. GDPR).
-
Data Prep
-
- Handle missing values, outliers, and inconsistencies in the data.
- Standardize data formats and units for consistency.
- Perform exploratory data analysis (EDA) to understand the characteristics, distributions, and patterns in the data.
-
Model Selection and Training
-
- Choose an appropriate Generative AI model architecture based on project requirements and data characteristics (e.g., GANs, VAEs, autoregressive models). Consider pre-trained models or architectures tailored to specific tasks.
- Train the selected model using the prepared dataset.
- Validate model outputs qualitatively and quantitatively. Conduct sensitivity analysis to understand model robustness.
-
Deployment Considerations
-
- Prepare the model for deployment in the business environment.
- Optimize model inference speed and resource requirements.
- Implement monitoring mechanisms to track model performance in production.
-
Documentation and Reporting
-
- Document all steps taken during data preparation, model development, and evaluation.
- Address concerns related to fairness, transparency, and privacy throughout the project lifecycle.
- Communicate findings and recommendations to stakeholders effectively for full transparency into processes.
Data preparation is a critical step for Generative AI because it ensures that the input data is of high quality, appropriately represented, and well-suited for training models to generate realistic, meaningful and ethically responsible outputs. By investing time and effort in data preparation, organizations can improve the performance, reliability, and ethical implications of their Generative AI applications.
Actian Data Preparation for GenAI
The Actian Data Platform comes with unified data integration, warehousing and visualization in a single platform. It includes a comprehensive set of capabilities for preprocessing, transformations, enrichment, normalization and serialization of structured, semi-structured and unstructured data such as JSON/XML, delimited files, RDBMS, JDBC/ODBC, HBase, Binary, ORC, ARFF, Parquet and Avro.
At Actian, our mission is to enable data engineers, data scientists and data analysts to work with high-quality, reliable data, no matter where it lives. We believe that when data teams focus on delivering comprehensive and trusted data pipelines, business leaders can truly benefit from groundbreaking technologies, such as GenAI.
Book a demo to see how Actian can help automate data preparation tasks in a robust, scalable, price-performant way.
Meet Our Team at the Gartner Data & Analytics Summit 2024
Join us for Gartner Data & Analytics Summit 2024, March 11 – 13, in Orlando, FL., where you’ll receive a step-by-step guide on readying your data for GenAI adoption. Check out our session, “Don’t Fall for the Hype: Prep Your Data for GenAI” on Thursday, March 12 at 1:10pm at the Dolphin Hotel, Atlantic Hall, Theater 3.
Subscribe to the Actian Blog
Subscribe to Actian’s blog to get data insights delivered right to you.
- Stay in the know – Get the latest in data analytics pushed directly to your inbox.
- Never miss a post – You’ll receive automatic email updates to let you know when new posts are live.
- It’s all up to you – Change your delivery preferences to suit your needs.
Subscribe
Subscribe to the Actian Blog
Subscribe to Actian’s blog to get data insights delivered right to you.
- Stay in the know – Get the latest in data analytics pushed directly to your inbox
- Never miss a post – You’ll receive automatic email updates to let you know when new posts are live
- It’s all up to you – Change your delivery preferences to suit your needs