How to Automatically Map Metadata Across Complex Ecosystems
Actian Corporation
April 5, 2025
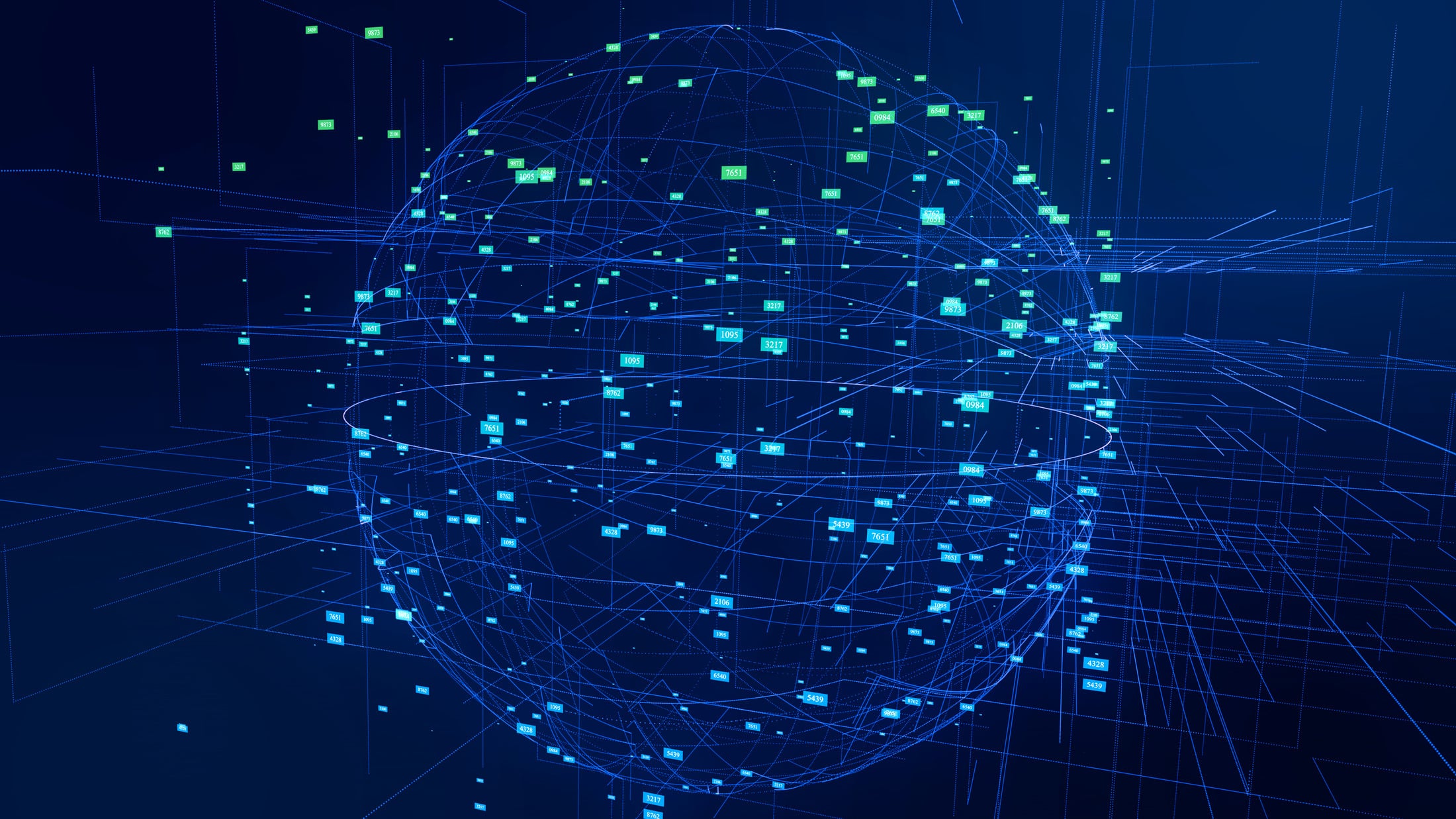
Organizations manage vast amounts of information daily across multiple systems, databases, and platforms. As data ecosystems grow in complexity, ensuring consistency, accuracy, and accessibility becomes increasingly challenging. This is where metadata management plays a crucial role. Effective metadata management enables organizations to track, organize, and govern data efficiently.
One of the most critical aspects of metadata management is metadata mapping, which ensures that data definitions, structures, and relationships remain aligned across various sources. Traditionally, mapping metadata required manual effort, making it labor-intensive and prone to errors. However, automation has transformed this process, allowing organizations to seamlessly map metadata across complex ecosystems.
In this article, we’ll explore how to automatically map metadata across large-scale enterprise environments, the benefits of automation, key challenges, and best practices to ensure accuracy and efficiency.
Understanding Metadata Management and Mapping
Metadata mapping is a part of an overarching metadata management program. As such, it’s important to understand the difference between the two concepts.
What is Metadata Management?
Metadata management refers to the administration of metadata, which includes defining, cataloging, and governing metadata across an organization. Metadata provides contextual information about data, such as its source, format, ownership, and relationships with other data assets.
Effective metadata management supports data governance, enhances discoverability, and ensures compliance with industry regulations.
What is Metadata Mapping?
Metadata mapping is the process of aligning metadata fields across different systems to ensure consistency and interoperability. It enables seamless data integration, transformation, and migration by defining relationships between different metadata elements.
For example, an organization may need to map customer data from a CRM system to a data warehouse while ensuring that attributes such as “Customer ID” and “Client Identifier” are correctly linked.
Challenges of Metadata Mapping in Complex Ecosystems
Managing metadata across a single database or application is relatively straightforward, but modern organizations operate within complex ecosystems that include:
- Multiple databases (SQL, NoSQL, data warehouses, cloud storage).
- Diverse applications (ERP, CRM, HR systems, BI tools).
- Hybrid and multi-cloud environments.
- Unstructured and structured data sources.
Some key challenges of metadata mapping in such environments are listed below.
1. Data Silos
Different business units often use disparate systems, leading to isolated metadata that lacks standardization. Known as data silos, these isolated environments make it difficult to transfer or use data across multiple sections of an organization.
2. Heterogeneous Data Formats
Metadata can exist in various formats (XML, JSON, CSV, relational databases, etc.), making it difficult to map fields accurately. Ideally, a company’s metadata management systems would account for these different formats.
3. Evolving Data Structures
Metadata definitions change over time due to system upgrades, business needs, or regulatory updates. Keeping mappings up to date is a consistent need that requires continuous monitoring.
4. Scalability Issues
Manually mapping metadata across thousands of data assets is time-consuming and more prone to error. Organizations can scale more easily and save time and money by automating the metadata mapping process.
5. Lack of Metadata Lineage
Understanding the origin and transformations of metadata is essential for compliance and data integrity. Without clear lineage tracking, errors can propagate across systems, and the source of these errors can be difficult to trace.
Automating Metadata Mapping: How it Works
Automated metadata mapping addresses these challenges by streamlining the process using machine learning (ML), artificial intelligence (AI), and metadata management platforms. Here’s how automation enhances metadata mapping.
Metadata Discovery and Classification
Automated tools scan various data sources to extract and classify metadata based on predefined rules. AI-powered engines can identify data types, formats, and structures automatically.
Schema Matching and Alignment
AI and machine learning algorithms compare metadata structures from different systems and suggest mappings based on similarities in data fields, patterns, and relationships.
Standardization
Automated tools enforce metadata governance policies to ensure that data elements conform to organizational standards, reducing inconsistencies.
Lineage and Traceability
Automation enables real-time tracking of metadata changes, providing a complete history of transformations, updates, and dependencies. This ensures transparency and compliance. It also makes error correction easier, as organizations can trace the source of any changes to the metadata.
Self-Learning and Continuous Improvement
AI-driven metadata management systems can learn from historical mappings, improving accuracy over time by recognizing common patterns and user-defined corrections.
Integration With Data Catalogs
Modern metadata mapping tools integrate with data catalogs, allowing users to search and retrieve metadata information efficiently. This enhances data discoverability and usability across the organization.
Benefits of Automating Metadata Mapping
Implementing automated metadata mapping provides organizations with several advantages.
1. Enhanced Accuracy and Consistency
Automation reduces human errors, ensuring that metadata mappings remain consistent across multiple systems.
2. Increased Efficiency and Scalability
Organizations can process and align metadata at scale without relying on manual intervention. This is crucial for large enterprises managing extensive data ecosystems.
3. Improved Compliance and Governance
With real-time metadata lineage tracking, organizations can meet regulatory requirements, such as GDPR, HIPAA, and CCPA, by maintaining accurate metadata records.
4. Faster Data Integration and Migration
Automated mapping simplifies data integration efforts, accelerating ETL (Extract, Transform, Load) processes and system migrations. ETL metadata mapping is important because it adjusts the metadata of incoming datasets to be consistent when performing the ETL process.
5. Cost Savings
Reducing manual efforts and preventing metadata inconsistencies helps organizations save time and resources, leading to lower operational costs.
6. ODS Mapping
An Operational Data Store (ODS) is a centralized repository that integrates data from multiple transactional systems to provide a consolidated, real-time view for operational reporting and analysis. ODS metadata mapping helps ensure data consistency, accuracy, and usability across the ecosystem.
Best Practices for Implementing Automated Metadata Mapping
To successfully implement automated metadata mapping, organizations should follow these best practices:
1. Define Metadata Governance Policies
Establish clear metadata standards, naming conventions, and governance policies to maintain consistency. This makes it easier to move data from one location to another or quickly find a data asset when needed.
2. Leverage AI and Machine Learning
Choose metadata management tools that utilize AI and ML to automate discovery, classification, and mapping processes. Actian’s platform offers robust metadata management tools, allowing organizations’ data teams to spend more time extracting value from assets and less time mapping.
3. Utilize a Centralized Metadata Repository
Store metadata in a centralized repository to ensure accessibility, visibility, and control across the organization. For example, organizations might choose to store data in a data warehouse or data lake.
4. Implement Metadata Lineage Tracking
Ensure that automated tools provide detailed lineage tracking to maintain audit trails and compliance records. This is especially important if the organization deals with sensitive or highly regulated information like credit card transactions.
5. Integrate with Existing Data Management Platforms
Choose solutions that seamlessly integrate with data catalogs, data lakes, and business intelligence tools to maximize efficiency.
6. Conduct Regular Metadata Audits
Automate metadata quality checks to identify and resolve inconsistencies before they impact business operations. Companies may also want to schedule manual audits of metadata and data assets at least once per year.
7. Enable User Collaboration and Feedback
Encourage data stewards and business users to review and refine automated mappings to improve accuracy.
Automate Metadata Mapping to Extract the Most Value Out of Data
Automating metadata mapping is essential for organizations operating within complex, data-intensive ecosystems. By leveraging AI-powered metadata management tools, businesses can ensure accuracy, efficiency, and compliance while reducing manual workload.
As data environments continue to evolve, investing in automated metadata mapping solutions will provide long-term benefits. These solutions enable organizations to maximize data value, streamline operations, and maintain a strong data governance framework.
Schedule a tour of the Actian Data Intelligence Platform to learn how Actian’s tool can help organize, map, and enhance crucial data assets.
Subscribe to the Actian Blog
Subscribe to Actian’s blog to get data insights delivered right to you.
- Stay in the know – Get the latest in data analytics pushed directly to your inbox.
- Never miss a post – You’ll receive automatic email updates to let you know when new posts are live.
- It’s all up to you – Change your delivery preferences to suit your needs.