9 Ways to Maintain Data Quality
Actian Corporation
November 3, 2023
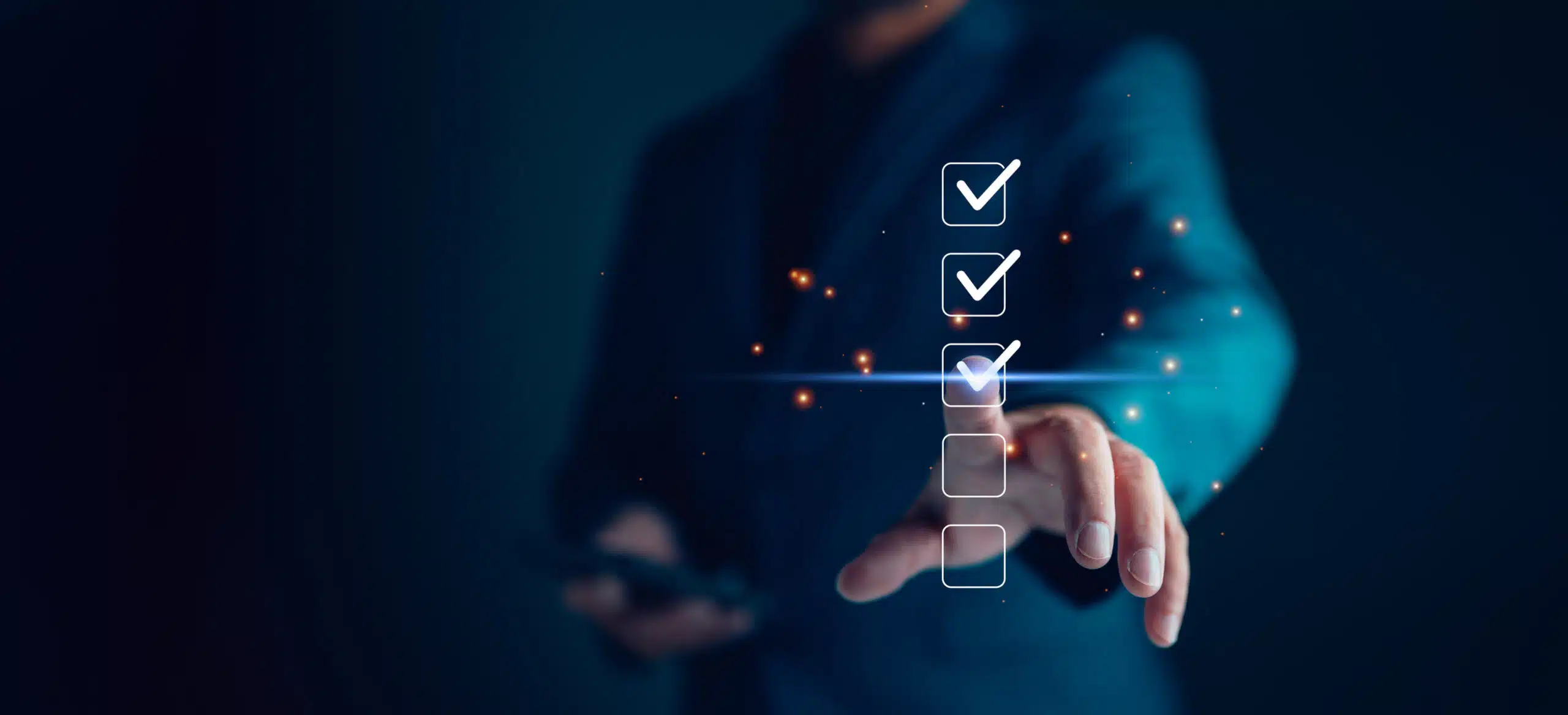
Data quality is essential to informing decisions, predicting and resolving problems, and enabling desired outcomes, but do you know how to maintain and deliver the quality your analysts and other data users need? A data management strategy is one essential component to ensure data meets your quality standards. Likewise, it’s important to understand and address common factors that reduce data quality.
At Actian, we define data quality management as “the mature processes, tools, and in-depth understanding of data you need to make decisions or solve problems to minimize risk and impact to your organization or customers.” The data must be accurate, current, complete, trusted, and usable by the various teams that need it.
Here are 9 ways to improve and maintain data quality:
1. Determine the Data Quality Standard You Need
You’ll need to define your standard for data quality. This standard should align with your business goals and anticipated uses to ensure the data meets your needs. The standard should also meet your data compliance and data governance requirements. Performing a data quality assessment lets you determine the current state of your data, then you can identify what needs to be improved to reach your data quality standard. When your data is trusted and meets the standard for its intended use, analysts and others will have confidence in the data and the analytics insights.
2. Create a Data Governance Framework
Data governance establishes the protocols and framework for maintaining data quality. It assigns the policies, processes, and roles within your organization to make sure data meets your quality standard for integrity, availability, and security. The framework also ensures your data meets compliance standards for regulated industries and for individuals’ personal data. A robust governance framework delivers quality data to all users, when and where it’s needed.
3. Implement Data Quality Tools
The right tools give you a modern approach to enabling data quality by automating processes for assessing data and identifying quality issues. The tools also help with essential processes such as profiling, cleansing, and standardizing data. Data management tools vary wildly in capabilities, so look for products that can provide a quick ‘at-a-glance’ view of data quality based on the rules you’ve established. These tools can also be integrated into data pipeline processes to automate data quality checks as data is ingested.
4. Profile Data to Identify Issues
Data profiling is essentially performing an audit to find quality issues. As Gartner notes, “Data profiling is a technology for discovering and investigating data quality issues, such as duplication, lack of consistency, and lack of accuracy and completeness.” Data profiling tools also look at data sources and metadata to uncover data errors. The process allows you to fix quality issues before the data is analyzed or integrated with other data, and it also allows you to solve problems to prevent them from reoccurring.
5. Cleanse Data to Address Inconsistencies
Gaps and inconsistencies can exist in datasets, which impact quality. Data that’s incorrect, incomplete, or has missing fields will not deliver the granular, trusted results users need. Data cleansing is a critical process that lets you find and fix inaccuracies, fill in missing information, and identify inconsistent data. The right approach to cleansing data helps ensure datasets are accurate, reliable, and complete.
6. Standardize Data into the Correct Format
Data standardization can be considered part of data cleansing. This process ensures data is in the required format for data users. It also makes sure you’re using a common format for all of your data for consistency and easier integration. Likewise, standardizing data makes it easier for you to perform data analytics and store the data because it’s in the most optimal format for your organization. Transforming the data into a usable, accessible, and shareable format ensures analysts and others can leverage it for maximum value.
7. Use Deduplication Processes to Eliminate Redundancies
Data redundancy, which results in multiple versions of the same data, is a common problem. Copies of data are made for backups, testing, specific uses, or other reasons. This can lead to data silos, which in turn increases costs by storing the same data several times. Data deduplication is the process that looks for and eliminates duplicate, or redundant, versions of data. The process identifies extra copies and deletes them so only a single instance of the dataset is stored. Deduplication helps with quality by eliminating data copies that can quickly become outdated, and it encourages analysts to use the current, verified data that’s available on a centralized data platform.
8. Train Employees to Recognize Quality Issues
Building a data-driven culture entails more than creating an environment in which everyone has access to and utilizes data. It also involves giving employees the proper tools and training them on best practices for maintaining data quality so they can identify issues and either fix them or report them. Many organizations have employees who focus on data stewardship, a role that’s responsible for the oversight and usage of data assets. Each department can have its own data steward to ensure data meets quality standards and that data governance policies are followed.
9. Monitor Data on an Ongoing Basis
Maintaining data quality is a continuous process. You can streamline much of it by using automated monitoring tools that routinely check and evaluate data quality, and identify any issues. When there is an issue, alerts are sent to notify the proper stakeholders to take corrective action. Continuous monitoring ensures that data maintains your quality standard as it’s shared and reused across the organization.
Making High-Quality Data Easy to Use and Analyze
Analysts, decision-makers, and others throughout the company must be able to trust the data in order to have confidence in the insights. Providing quality data is one way to establish that trust. Actian can help. We offer tools and expertise to help you identify and correct data anomalies to give you high-quality data that improves the effectiveness of your data-driven initiatives. We also make data easy. The Actian Data Platform simplifies how you connect, manage, and analyze data. This makes trusted data readily and easily available to everyone in your organization to accelerate your growth.
Additional Resources:
- Mastering Your Data With a Data Quality Management Framework
- Data Profiling and Data Quality: What You Need to Know
- The High Costs of Dirty Data – Why Data Profiling with DataConnect Version 12 Will Improve Your Business Outcomes
Subscribe to the Actian Blog
Subscribe to Actian’s blog to get data insights delivered right to you.
- Stay in the know – Get the latest in data analytics pushed directly to your inbox
- Never miss a post – You’ll receive automatic email updates to let you know when new posts are live
- It’s all up to you – Change your delivery preferences to suit your needs