Everything You Need to Know About Data Ops
Actian Corporation
March 26, 2020
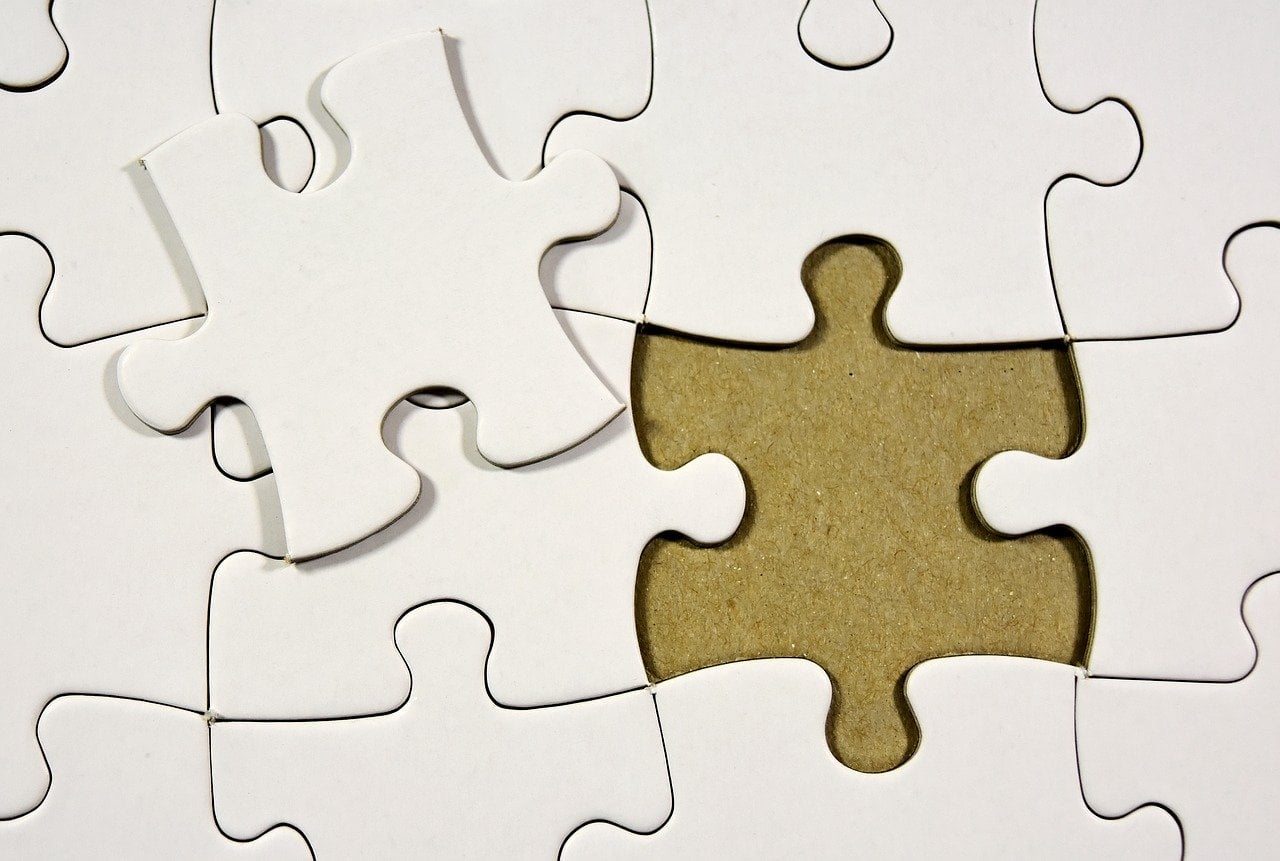
“Within the next year, the number of data and analytics experts in business units will grow at three times the rate of experts in IT departments, which will force companies to rethink their organizational models and skill sets” – Gartner, 2020.
Data and Analytics teams are becoming more and more essential in supporting various complex business processes, and many are challenged with scaling the work they do in delivering data to support their use cases. The pressure to deliver faster and with higher quality is causing data & analytics leaders to rethink how their teams are organized.
Where traditional waterfall models were implemented and used in enterprises in the past, these methodologies are now proving to be too long, too siloed, and too overwhelming.
This is where Data Ops steps in: a more agile, collaborative, and change-friendly approach for managing data pipelines.
Data Ops Definition
Gartner defines Data Ops as being a “collaborative data management practice focused on improving the communication, integration and automation of data flows between data managers and data consumers across an organization”. Basically, it makes life easier for data users.
Similar to how DevOps, a set of practices that combines software development (Dev) and information-technology operations (Ops), changed the way we deliver software, DataOps uses the same methodologies for teams building data products.
While both agile frameworks, DataOps requires the coordination of data and anyone that works with data across the entire enterprise.
Specifically, data and analytics leaders should implement these key approaches proven to deliver significant value for organizations:
- Deployment Frequency Increase: Shifting towards a more rapid and continuous delivery methodology enables organizations to reduce the time to market.
- Automated Testing: Removing time-consuming, manual testing enables higher quality data deliveries.
- Metadata Control: Tracking and reporting metadata across all consumers in the data pipeline ensures better change management and avoids errors.
- Monitoring: tracking data behavior and the usage of the pipeline enables more rapid identification on both flawed – that needs to be corrected – and good quality data for new capabilities.
- Constant Collaboration: communication between data stakeholders on data is essential for faster data delivery.
Who is Involved in Data Ops?
Given the importance of data and analytics use cases today, the roles involved in successful data project delivery are more numerous and more distributed than ever before. Ranging from data science teams to people outside of IT, a large number of roles are involved:
- Business analysts
- Data architects.
- Data engineers.
- Data stewards.
- Data scientists.
- Data product managers.
- Machine Learning developers.
- Database administrators.
As mentioned above, a Data Ops approach requires fluid communication and collaboration across these roles. Each collaborator needs to understand what others expect of them, what others produce, and must have a shared understanding of the goals of the data pipelines they are creating and evolving.
Creating channels through which these roles can work together, such as a collaboration tool, or metadata management solution, is the starting point.
Subscribe to the Actian Blog
Subscribe to Actian’s blog to get data insights delivered right to you.
- Stay in the know – Get the latest in data analytics pushed directly to your inbox.
- Never miss a post – You’ll receive automatic email updates to let you know when new posts are live.
- It’s all up to you – Change your delivery preferences to suit your needs.