Data Ops Rules to Avoid Data Oops
Actian Corporation
April 6, 2020
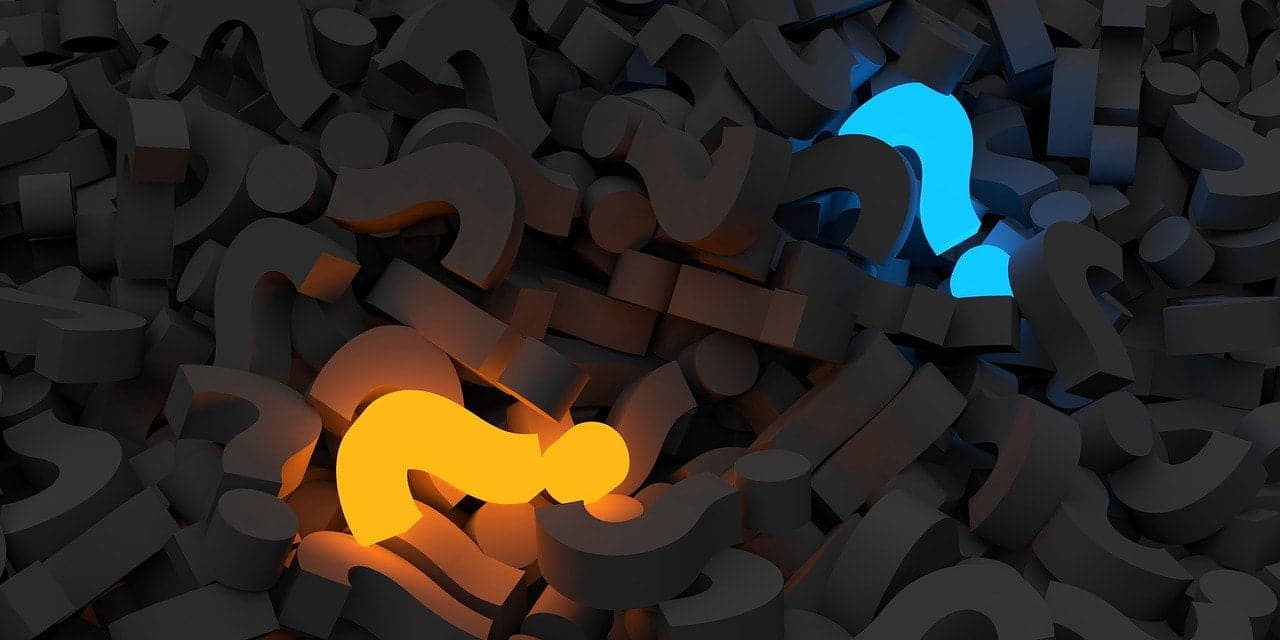
Data Ops is a new way to address the deployment of data and analytics solutions.
The success of this methodology is based on techniques that promote faster, more flexible, and more reliable data delivery. To deliver on this promise, let’s take a moment and analyze this sentence: “the focus is not just on building the systems right, but also building the right systems”.
Many different definitions, interpretations, and publications address DataOps as a concept, but it is much more than just that. It is a way of understanding, discovering, producing analysis, and creating actionable intelligence with data. In a changing world that revolves around data, latencies in data products or their analysis are no longer acceptable.
The entire organization must be put to work to support the deployment and improvement of data and analysis projects!
Data Oops definition
The concept of DataOps emerged in response to the challenges of failing data systems and failing data project implementations, but also the fragility, friction, or even fear when it comes to the use of data. If you are experiencing this situation, then don’t look too far…you are in the middle of a Data Oops!
In this context of Data Oops, you will agree that your data teams are struggling to achieve the speed and reliability of directed projects.
The main reasons are that companies have too many roles, are too complex, and are constantly changing requirements or objectives, making tasks difficult to frame and deliver.
This complexity is exacerbated by a lack of confidence in data, even to the point of “fearing” it. This occurs when we observe limited or inconsistent coordination between the different roles involved in the construction, deployment, and maintenance of data flows. We are convinced that an organization that does not know its data is doomed to fail.
How to Succeed in your DataOps
Simply put, DataOps is a collaborative data management practice that aims to improve communication, integration and automation of data flows between data managers and data consumers within an organization. It is based on the alignment of objectives confronted by results. DataOps accepts failure and is built through continuous experimentation.
Here’s a list of principles for successful DataOps:
- Learn from DevOps, through their techniques for developing and deploying agile applications in your data and analysis work.
- Identify quantifiable, measurable and achievable business objectives. You will then be able to communicate more regularly, progress towards a common goal and adjust more easily.
- Start by identifying and mapping your data (type, format, who, when, where, why, etc.) using data catalog solutions.
- Encourage collaboration between different data stakeholders by providing communication channels and solutions for sharing metadata.
- Take care of your data, as it may produce value at any given time. Clean it, catalog it, and make it a part of your enterprise’s key assets, whether it is valuable now or not.
- A model may work well once, but not on the next batch of data. Over-specifying and over-engineering a model will likely not be applicable to previously unseen data or for new circumstances in which the model will be deployed.
- Maximize your chances of success of introducing a DataOps approach by selecting data and analysis projects that are struggling due to a lack of collaboration or are struggling to keep pace. They will allow you to better demonstrate its value.
- Keep it agile, short designed, develop, test, release, and repeat! Keep it lean and build on incremental changes. Continuous improvement is found when a culture of experimentation is encouraged and when people learn from their failures. Remember, data science is still science!
In Summary, What are the Benefits of DataOps?
DataOps helps your business move at the speed of data – keeping pace to deliver the right data. It focuses data activities to be aligned with business objectives, and not on the analytic inputs (big data hype). DataOps also focuses on delivering value from all your data activities, from even the smallest of these can inspire cultural changes needed for other implementations to come.
Adopting DataOps in a culture of experimentation is good data practice and empowers the innovators across the organization to start small and scale fast. It is the path to good business practices.
Subscribe to the Actian Blog
Subscribe to Actian’s blog to get data insights delivered right to you.
- Stay in the know – Get the latest in data analytics pushed directly to your inbox.
- Never miss a post – You’ll receive automatic email updates to let you know when new posts are live.
- It’s all up to you – Change your delivery preferences to suit your needs.