A Guide to Data Quality Assurance
Actian Corporation
April 17, 2025
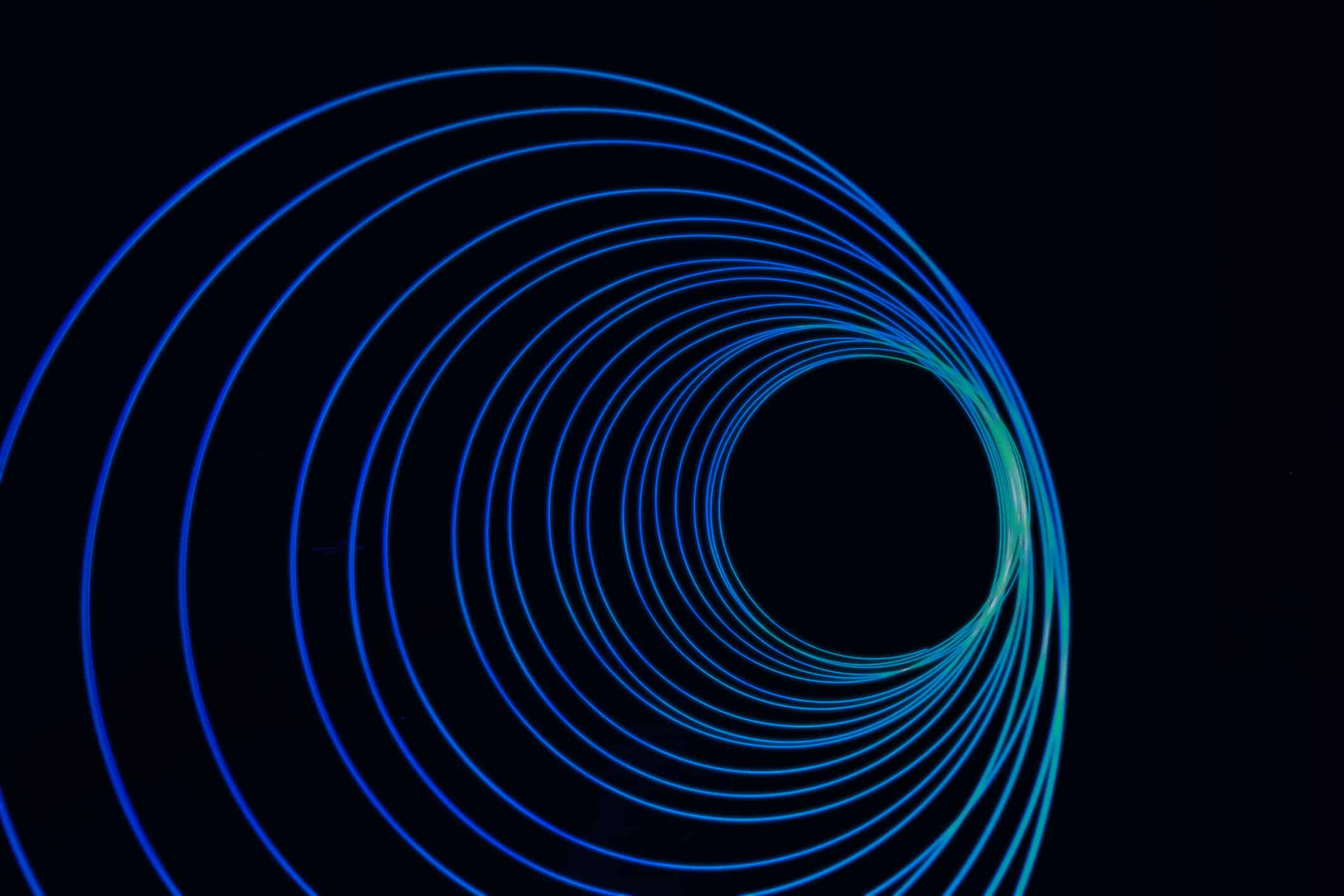
The importance of high-quality data cannot be overstated. Data is the foundation for decision-making, analytics, machine learning models, and operational processes. However, the usefulness of data is heavily dependent on its quality. This is where data quality assurance (DQA) can help by ensuring that the data used by organizations is accurate, consistent, and reliable.
What is Data Quality Assurance (DQA)?
Data quality assurance is the process of monitoring, evaluating, and improving data quality to ensure that it meets predefined standards and is suitable for its intended purposes. It involves various techniques, methodologies, and tools that ensure the data used within an organization is both accurate and consistent, ultimately leading to better decision-making, improved efficiency, and more reliable insights.
DQA is not just about identifying and correcting errors in data, but also about preventing these errors from occurring in the first place. It’s a continuous process that requires proactive planning, monitoring, and evaluation at every stage of the data lifecycle.
Key Elements of Data Quality
Data quality can be assessed through several key dimensions. These include:
- Accuracy: Ensuring that the data is correct and free from errors. Accuracy is crucial for making informed decisions and conducting reliable analyses.
- Consistency: Data should be consistent across different systems, databases, and applications. Inconsistencies can arise when data is entered or updated in multiple places, leading to discrepancies.
- Completeness: Data should be comprehensive and not missing critical information. Incomplete data can lead to skewed analysis and missed opportunities.
- Timeliness: Data should be up-to-date and available when needed. Outdated data may lead to decisions based on old or irrelevant information.
- Validity: Data must conform to predefined standards, formats, and rules. Invalid data can result in inaccurate analyses or even system failures.
- Uniqueness: Ensuring there are no duplicate records or redundant entries that could impact the quality of the data set.
- Relevance: Data should be pertinent to the specific objectives and context in which it is being used. Irrelevant data can clutter systems and make it harder to extract valuable insights.
Importance of Data Quality Assurance
Ensuring data quality is essential for several reasons. Let’s take a look at a few of them.
Improved Decision-Making
High-quality data enables organizations to make well-informed decisions. Whether it’s in business strategy, financial forecasting, or operational efficiency, decisions based on accurate, timely, and relevant data are more likely to succeed.
Operational Efficiency
Poor data quality can lead to inefficiencies, redundancies, and increased operational costs. For example, DQA can identify and rectify errors early, preventing them from cascading into larger operational issues. This reduces costs associated with correcting mistakes, such as billing errors or customer refunds, and avoids inefficiencies caused by poor data quality.
Regulatory Compliance
Many industries, such as healthcare and finance, must adhere to strict regulatory requirements regarding data accuracy and integrity. DQA helps organizations maintain compliance and avoid costly penalties.
Customer Trust
In an era where customer data is a critical asset, maintaining high data quality is key to building trust. If an organization continuously provides correct and timely information, customers are more likely to rely on and engage with the brand.
Enhanced Analytics and Reporting
Data quality directly impacts the effectiveness of data analysis and reporting. Clean, consistent, and accurate data ensures that reports and business intelligence tools provide reliable insights to drive positive outcomes.
Best Practices for Data Quality Assurance
To ensure effective data quality assurance, organizations should implement the following best practices:
Establish Clear Data Governance
Create a comprehensive data governance framework that outlines standards, policies, and procedures for managing data across the organization. This ensures that everyone follows the same guidelines and can maintain data quality throughout its lifecycle.
Implement Data Validation Rules
Use validation techniques at the point of data entry to ensure that only high-quality data enters the system. For example, use constraints, drop-down lists, and format checks to prevent invalid or incomplete data from being recorded. Additionally, data quality solutions can be added to the data pipeline to isolate invalid data for further remediation.
Regular Data Audits
Conduct regular data audits to identify discrepancies, errors, and inconsistencies in your data. Automated tools can help to track changes and monitor data quality in real time.
Use Data Profiling and Cleansing Tools
Data profiling tools help organizations to assess the quality of data in terms of structure, patterns, and completeness. Once data issues are identified, data cleansing tools can remove duplicates, correct errors, and standardize data for consistency.
Implement Training and Awareness
Ensure that all employees who handle data are trained in best practices for data entry and management. This includes understanding the importance of accurate data and how to spot potential issues.
Monitor Data Quality Continuously
Data quality is not a one-time effort. Continuous monitoring of data quality helps to identify emerging issues and resolve them before they can have a significant impact on the organization.
Collaborate Across Departments
DQA is not just an IT or data team responsibility. Data quality should be a cross-departmental effort. Engaging teams from operations, finance, marketing, and other departments ensures that data quality needs are met across the organization.
Ensure Data Accuracy With Actian’s Data Intelligence Platform
Data quality assurance is essential for organizations to ensure their data is accurate, consistent, and reliable. By implementing best practices, utilizing the right tools, and establishing a robust data governance framework.
Organization can ensure that their data is accurate by partnering with Actian. Our data intelligence platform helps organizations make better business decisions by enabling data users to quickly detect and act on a dataset’s quality to uncover its trustworthiness before an issue arises. Benefit from advanced metadata management to simplify search, exploration, governance, and compliance – all in one unified platform.
Subscribe to the Actian Blog
Subscribe to Actian’s blog to get data insights delivered right to you.
- Stay in the know – Get the latest in data analytics pushed directly to your inbox.
- Never miss a post – You’ll receive automatic email updates to let you know when new posts are live.
- It’s all up to you – Change your delivery preferences to suit your needs.