Data Mesh vs. Data Fabric: Which One Should I Choose?
Nick Johnson
April 8, 2025
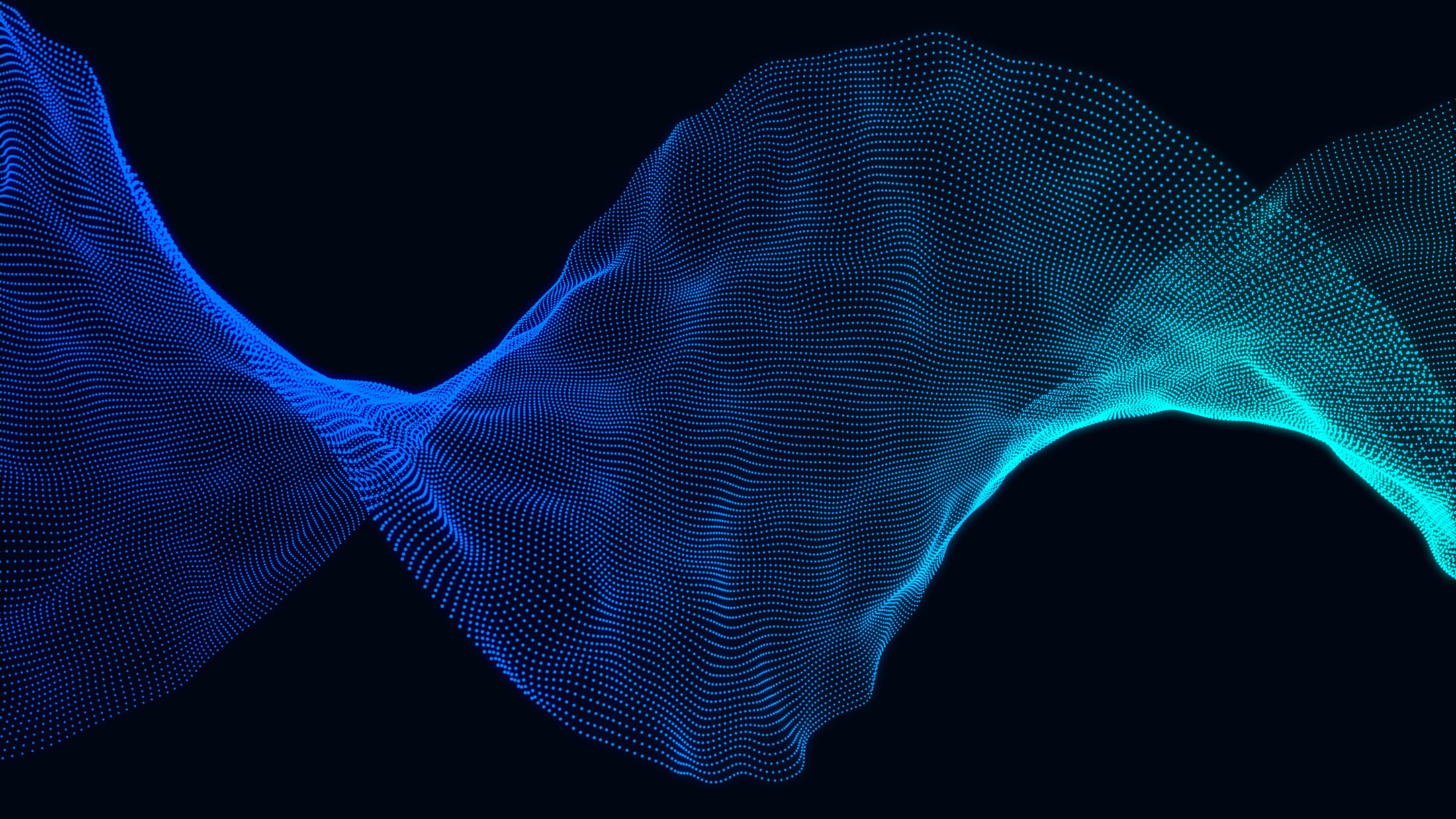
Centralized or Decentralized Data Governance?
Should you centralize your data management for better control or decentralize it for more agility? The answer might not be as straightforward as it seems.
Data fabric and data mesh offer two distinct approaches to managing and sharing data within an enterprise. They are often discussed in opposition to one another: data fabric emphasizes centralized data access, governance, and security, while data mesh promotes a more democratized and decentralized model.
Both approaches aim to address common data challenges, including:
- Ensuring that the right data reaches the right people at the right time to maximize productivity.
- Guaranteeing data accuracy, consistency, and completeness.
- Protecting sensitive data from unauthorized access.
Neither model is a perfect solution for most organizations, as each has its strengths and limitations. However, a large opportunity exists to combine elements of both approaches to create a governance model that best suits the needs of your business.
What is Data Fabric?
Data fabric is a unified semantic layer that integrates disparate data sources and applications. It enables reusable data pipelines, data lakehouse storage, and metadata management. This approach improves interoperability, streamlines data access, and centralizes security and compliance.
However, overreliance on a single architecture paradigm may lead to costly dependencies, increased complexity, and scalability challenges that prevent innovation. The “data platform” construct is a practical example of the data fabric concept, but when applied rigidly, it can create bottlenecks that can be difficult to overcome.
What is Data Mesh?
Data mesh is a decentralized data architecture and operating model that enables domain-specific teams to assume ownership of their data and treat it as a product. By fostering a federated governance model, data mesh adheres to enterprise-wide policy standards while empowering teams to make autonomous decisions. This model cultivates a culture of accountability, ensuring data quality at the source.
However, aligning diverse governance standards across multiple domains can lead to inconsistencies in data definitions and security risks if not managed cohesively. The proper execution of a data mesh strategy requires a strong governance framework to maintain interoperability across teams.
Lessons From the Era of “Big Data”
Historically, large enterprises leaned toward data fabric as cloud providers like Microsoft, Amazon, Google, Snowflake, and Databricks promoted centralized big data analytics. The cloud data warehouse became the designated “single source of truth,” intended to standardize and govern vast amounts of structured, semi-structured, and unstructured data.
However, as data volumes exploded, data teams struggled to maintain clarity and consistency. Many teams ended up without access to well-governed data, resorting to unmanaged spreadsheets or relying on IT departments, creating further delays and complexity. The promise of big data analytics transformed into a flood of messy, unmanageable data.
Is a Hybrid Approach the Future?
To address the challenges of big data, organizations are increasingly considering a hybrid approach combining data fabric and data mesh principles. According to Gartner’s 2024 Evolution of Data Management Survey, 22% of organizations have implemented data fabric, 26% have adopted data mesh, and 13% already utilize both.1 The number of organizations that adopt a hybrid approach is expected to grow over the next few years.
A hybrid approach leverages the complementary strengths of both data fabric and data mesh. Gartner predicts: “By 2028, 80% of autonomous data products supporting ‘AI-Ready data’ use cases will emerge from a fabric and mesh complementary architecture.”2 Here, data fabric acts as the foundational data management infrastructure, while data mesh provides the delivery framework for high-quality data products.
Why a Hybrid Approach Matters
A hybrid model ensures strong data stewardship by unifying data design and governance (data fabric) while maintaining agility and domain-specific context (data mesh). This approach transforms central data teams from gatekeepers to mediators who support domain-specific teams in maintaining data quality and consistency. Centralized governance establishes enterprise-wide standards, while federated autonomy ensures domain expertise shapes data usage effectively.
Cross-functional collaboration remains essential in a hybrid data architecture. Organizations must balance centralized governance principles with domain-specific insights to ensure data products remain discoverable, trusted, and easy to access.
Choosing the Right Approach
Your organization’s choice of data governance strategy should reflect factors such as company size, diversity and complexity of data sources, departmental structure, and regulatory requirements.
Many growing companies succeed by implementing centralized governance first, then expanding principles to domain-specific areas. However, some large, complex enterprises may need to collaborate initially with domain teams to establish governance standards effectively, given existing data volumes and complexity.
Ultimately, the hybrid approach is the desired end state, offering the flexibility and control necessary to harness your data and operate at peak performance.
1 2024 Gartner Evolution of Data Management Survey, Gartner, 2024
2 How Data Leaders can Settle the Data Fabric and Mesh Debate, Gartner, 2025
Subscribe to the Actian Blog
Subscribe to Actian’s blog to get data insights delivered right to you.
- Stay in the know – Get the latest in data analytics pushed directly to your inbox.
- Never miss a post – You’ll receive automatic email updates to let you know when new posts are live.
- It’s all up to you – Change your delivery preferences to suit your needs.