Why Data Scientists and Developers Need More Than a Data Lake
Teresa Wingfield
August 15, 2023
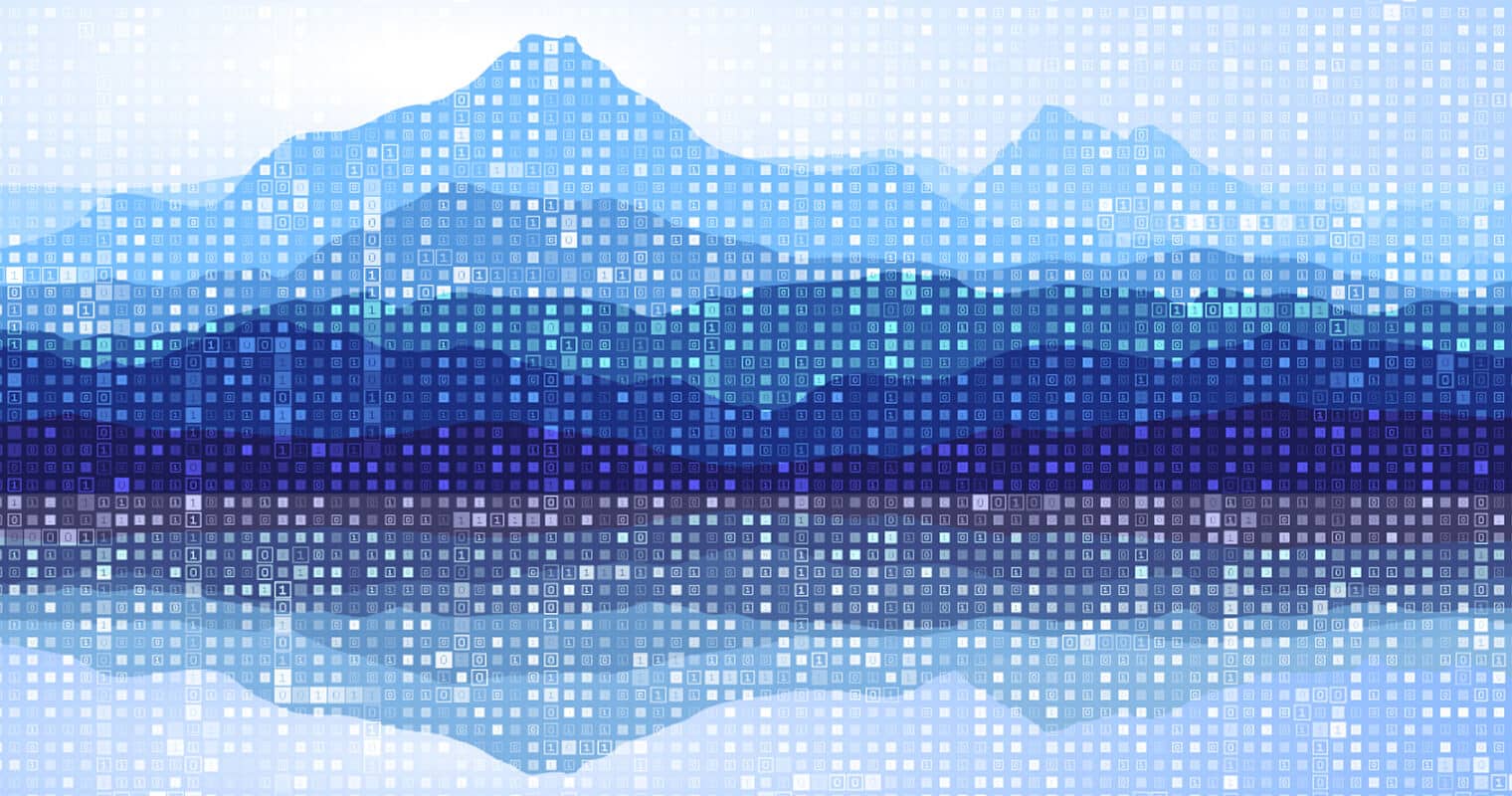
As organizations strive to get more value from the data they collect, it has become increasingly important that data scientists and developers have easy access to information collected from multiple sources in various sizes and formats. For many businesses, creating a data lake has become the first step in this process, forming a useful repository for large amounts of data that can be analyzed and tested down the road.
However, while these repositories can create new opportunities for extracting business insights, data lakes on their own may not always be the answer. While they provide a centralized location for all of an organization’s data, they can also be challenging to manage and control.
Why Are Data Lakes Useful to Organizations?
When organizations begin extracting raw, unstructured data from multiple sources, they must have a sustainable and organized format in place for storage. One of the benefits of using a data lake is that it allows organizations to keep all of their data in one place. This can be especially helpful for companies with multiple silos of information scattered across different departments or locations. But it’s also important to note that data lakes are often used for very unstructured data and can easily become a data swap since data can often lack any context or structure to be useful.
Another benefit of data lakes is that they can be used to support a variety of analytics workloads. For example, data scientists and developers can use data lakes for real-time streaming analytics, machine learning models, and AI.
Data lakes are also relatively easy and inexpensive to establish. Because they can store data in its rawest form, organizations don’t need to spend time and money on ETL (extract, transform, load) processes.
What Are the Limitations of Data Lakes?
So, if data lakes are so great, why do data scientists and developers still need to look for other solutions when working with data?
One of the biggest challenges with data lakes is that they can be challenging to manage. Because data lakes store all types of data, it can be hard to keep track of everything there. It’s also challenging to control access to the data and ensure that only authorized users can view or modify it.
A predominant issue with data lakes is that they can often contain a lot of duplicate or low-quality data. This can make it time-consuming and difficult for data scientists and developers to find the specific information they need. And this can be a particular problem if the data lake has not been adequately curated.
Are Data Lakes Enough for Businesses?
Although data lakes are an excellent solution for housing unstructured data, they are often not enough for data scientists and developers when extracting all the relevant insights contained in the information. This is due to the unstructured formatting of data lakes, making the integrity of the analysis questionable and potentially inaccurate without considerable data cleansing.
Data warehouses, on the other hand, can provide a better solution for providing analysis and business insights. The information held in data warehouses is typically normalized, meaning it is cleansed, consistent, and organized into tables with well-defined relationships between them. This makes it easier to write SQL queries against the data and can be more reliable when ensuring accuracy and overall data integrity.
However, while data warehouses store data in more of a “ready” state for analysis, this doesn’t mean that data lakes are absolute for data scientists and developers. In fact, data lakes are regularly used for many experimental processes, such as data discovery and machine learning. Being able to store data in raw and unstructured formats can give data scientists much more freedom when exploring the data for insights, rather than being confined to work with normalized and structured data.
Understanding the Connection Between Data Lakes and Data Warehouses
Although data lakes and data warehouses may be different, it’s important to note that each of them is not mutually exclusive. For modern businesses, there is a convergence of these two technologies, with many organizations using both data lakes and data warehouses to manage their big data.
Data lakes and data warehouses can actually complement each other well. A data warehouse can act as the single source of truth for an organization. Meanwhile, a data lake can be used to store all of the organization’s data, including data from sources that aren’t yet well understood or trusted enough to be placed in the data warehouse. In fact, ETL (Extract, Transform, Load) tools are used for this very purpose, automatically redirecting raw, unstructured information from the data lake and organizing it efficiently in a data warehouse.
It’s important for businesses to discover how they can use data lakes and data warehouses collectively as opposed to staying focused on a particular format. While each project may have its own needs when it comes to data storage and analysis, by understanding the benefits and trade-offs of each data platform, companies can make more informed decisions about how to use them together and get the most out of their data collection efforts.
Subscribe to the Actian Blog
Subscribe to Actian’s blog to get data insights delivered right to you.
- Stay in the know – Get the latest in data analytics pushed directly to your inbox.
- Never miss a post – You’ll receive automatic email updates to let you know when new posts are live.
- It’s all up to you – Change your delivery preferences to suit your needs.
Subscribe
Subscribe to the Actian Blog
Subscribe to Actian’s blog to get data insights delivered right to you.
- Stay in the know – Get the latest in data analytics pushed directly to your inbox
- Never miss a post – You’ll receive automatic email updates to let you know when new posts are live
- It’s all up to you – Change your delivery preferences to suit your needs