Fact: Data Quality Management Increases ROI
Decision-making and problem-solving are based on data you receive and interpret to achieve your corporate vision, help staff or customers, or address an issue. Before COVID-19, Gartner estimated that the annual financial impact of poor data quality was in the region of $15m.
Data Quality Management (DQM) impacts the ability to create a Master Data Management (MDM) strategy. Gartner and ISACA believe that data quality must be a board-level recurring topic and concern. Only senior leadership benefitting from data quality management tools and dashboards can create the culture and behaviors that stop data from becoming a dangerous liability instead of a strategic asset.
What is Data Quality Management?
Data Quality Management is the mature processes, tools, and in-depth understanding of data you need to make decisions or solve problems to minimize risk and impact to your organization or customers. You can have data that is of high quality and still achieve poor business outcomes. DQM is the practice of using that data to serve your purposes with flexibility and agility. To do this, you must assess what data you have today and the processes and tools that use or support data against accuracy, completeness, consistency, and timeliness measures.
DQM and MDM practices of data acquisition, use, and storage are part of everyone’s role, including vendors. This makes data a holistic asset, by which we mean that data is the input and output of every task and transaction performed by your business. As such, the quality of your data will be improved by following best industry practices (DQM) which begin with designing a view of data flow and use (MDM).
Sound DQM and MDM practices will help overcome:
- Lack of trust in data causing employees to create their versions of data (non-standard across multiple Excel spreadsheets and data tables).
- Insufficient data underpinning bad decisions.
- The increased cost of data management and storage.
- Lack of uniformity in data use or feel, causing complicated use of applications.
- Creating levels of unacceptable risk or potential reputational damage as data is incorrectly managed.
- Communication and collaboration silos caused by deficient or misunderstood data.
- Inability to react to volatile market changes or crises (e.g., COVID-19).
- Failure to introduce digital practices prevents cross-departmental usage of non-structured data.
Simply put: data management is a holistic practice affecting every aspect of your organization. Your Master Data Management processes and model will mature as you introduce a Data Quality Management framework.
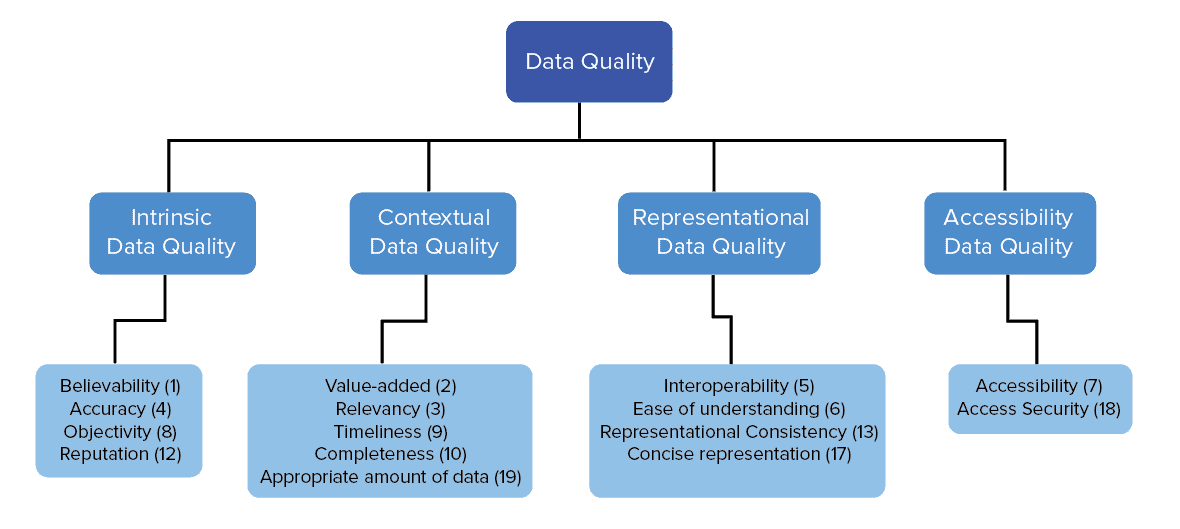
Another suggestion would be to look at the frameworks and tools offered by cloud providers, such as Azure Purview. This tool highlights the lineage of your data from inception to archival or deletion. In this way, you build a set of data sources (data warehouses, data lakes) that are trusted and support all of your governance and audit scenarios. The best suggestion is to learn from data management and integration consultants that can help introduce tools and processes that integrate, monitor, verify, archive and remove your data across your defined master and quality data management models.
Attributes of Data Quality Management
Quality data management is what makes your business healthy. As mentioned earlier, DQM has the following attributes:
- Completeness: Any missing fields or missing information?
- Validity: Does the data match its need and use?
- Uniqueness: Are you relying on the correct set of data and not a bunch of redundant but outdated information sets?
- Consistency: Is the same information available to all concerned and does not vary by application of business domain?
- Timeliness or Age: Does the data represent the most accurate and available information available at the time of use?
- Accuracy: Are the data values as expected?
- Integrity: Does the data qualify as usable against your data quality governance standards?
Data Quality Management Framework and Best Practices
Keeping in mind the attributes of data quality and its formulation in a master data system will help create the structure of your framework. DQM and MDM frameworks support the rules and measures of data quality that ensure your confidence in the data feeding decisions and problems are sound.
An MDM framework will guide your data quality governance policies and processes, along with the guardrail metrics and rules to help staff, IT, and vendors keep your data safe, secure, and usable. As you build the framework, consider:
- Accountability: Who will be the leading purveyor of your data strategy and governance? Many are creating a new role of Chief Data Officer (CDO) to perform this function.
- Transparency: How will the rules of data be shared? What feedback will you gather to influence changes to policies?
- Compliance: How will you know that policies and standards are followed? What will be the penalty for violating a data policy? How will you ensure that your policies meet regulatory mandates?
- Protection: What will you do to archive, cleanse, modify, secure, backup, recover and delete data? How do you know you have data issues? What monitoring and alerting must you have for incoming data sources (from the internet, for instance) or outgoing (is data being transferred illegally, or have you been hacked)?
An excellent model to adopt and adapt is from professors Richard Wang and Diane Strong Beyond Accuracy: What Data Quality means to economic consumers.
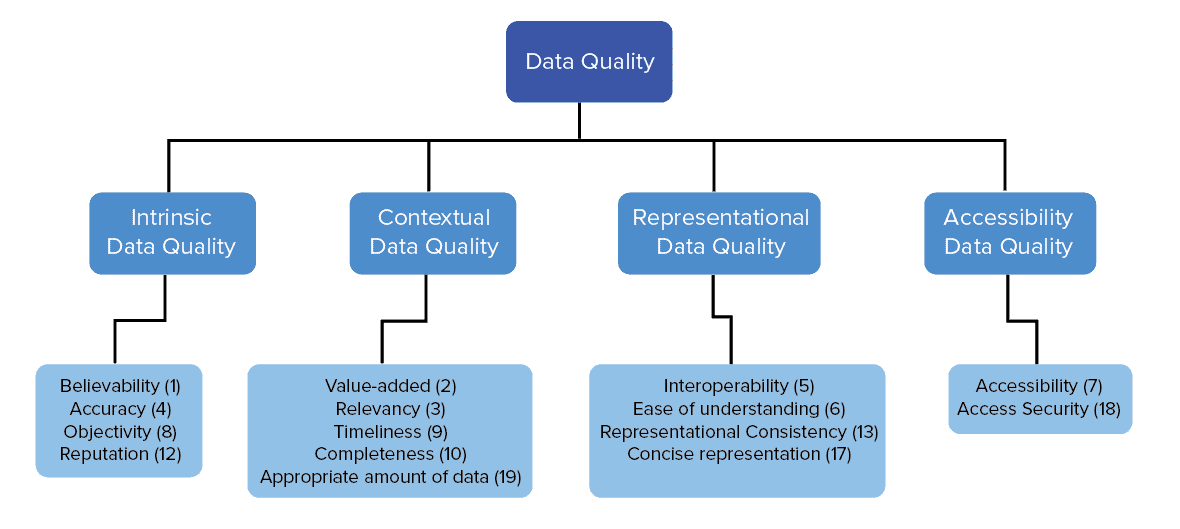
Another suggestion would be to look at the frameworks and tools offered by cloud providers, such as Azure Purview. This tool highlights the lineage of your data from inception to archival or deletion. In this way, you build a set of data sources (data warehouses, data lakes) that are trusted and support all of your governance and audit scenarios. The best suggestion is to learn from data management and integration consultants that can help introduce tools and processes that integrate, monitor, verify, archive and remove your data across your defined master and quality data management models.
Where Do You Begin?
The first question to ask is what is the data we use? Using a simple version of mapping your work (value stream mapping) can expose all the various data in your organization, where and why it is used, and how it is managed. Without an assessment, the master data management model you create will not be fit for purpose which will impact any quality metrics you hoped to achieve. After conferring with your development teams and data management partners, careful design will help you avoid the traps of poor data management practices.
Your design will lead to a series of pilots as you begin to assess the data flowing across your organization in terms of quality and control. The pilot outcomes will help structure your Data Quality Governance Framework. This framework will help development teams and users on how to best use and benefit from data as an information source via tools, templates, policies, training and monitoring which encompass your Master Data Management framework for each dataset or source within your business.
The CDO or data owners within each business function should be part of the governance team and help implement access rights and management by becoming data stewards. Remember that IT should only act as the facilitator of creating data frameworks, warehouses, or lakes. The business domain data stewards own (are accountable) for their data, use, and maintenance.
Data is your critical asset. Your pilots should begin by improving the quality and control of your critical or most often used or updated data. Every data source should use a pilot (learn) approach based against your data governance, quality and master data management frameworks. In this way your agility and flexibility will be maintained, and as you mature your practices, will help lead to a more viable digital business capable of survival in a volatile global market.
Data Quality Activities and Lifecycle
These primary activities will appropriately govern your data quality:
- Acquisition: Getting the data from a source, manual entry or as application output.
- Analyze: Deciding if the data meets quality standards for use, usually performed via an application requiring the data.
- Cleansing: Removing any data element not needed, keeping the size to a minimum.
- Enrichment: Adding more data to create a set of information such as multiple data sources to create financial reports.
- Monitoring and Alerting: The primary activity to maintain safety, security, and compliance.
- Repair: If a dataset becomes corrupt, this step will help in correcting issues.
- Archival or Deletion: Saving data against agreed requirements.
- Reporting: This could be the regular reports or screens your enterprise uses, but in terms of data quality, this is the step that reports and logs data issues, how they are addressed and whether the problems repeat.
Data Quality Management Process
Data Quality Management processes will differ based on the use of data per business area. This is why your framework needs to be holistic in vision but tactically provide guardrails such that every team or activity can strive for the quality needed to ensure safety, ease, compliance, cost, and competitiveness. The same concept applies as you build your Master Data Management framework highlighting the basic rules for data acquisition, use, storage, and access.
Enterprise Data Quality Management
It is a complex but necessary set of actions to create a robust data quality and master data framework. Without the commitment and senior leadership support and funding, your data quality will be suspect and might cause you to become vulnerable. Therefore, looking at data from the enterprise level and then working downwards is an excellent approach for ensuring that what is needed is of the quality required.
Shift-left from the customers you are serving or from the outcomes of internal work tasks to see if the data meets expectations. Product management, DevOps, Agile, and ITSM thinking can provide the basis for your enterprise data management policies, rules, and tools. Once in place, you can begin to broaden the scope of data usage to include customer behavior, competitor status, issues seen at other companies that you might be able to fulfill (first to market), and more.
Data profiling is like the detective work you see on TV shows. Is your data matching the rules needed, and if not, why not? These inconsistencies will be where you must cleanse and repair data as soon as practical. One way of implementing data profiling is by having an application or product-to-application product checker. Another is via a business glossary of data and terms used within your enterprise. Your data management consulting partner can provide templates and guide you as to how best to create and control your data such that it is always ready to be used by a task or underpin a decision based upon the outcome of data analysis.
Data Quality Metrics
Data quality metrics are the guardrails of data quality and MDM frameworks. The suggested ratios below form the standard data quality measures, but these can be influenced by other business metrics you currently apply.
- Ratio of Data to Errors: How many issues are there for each dataset, data warehouse, or data lake as seen by application errors or security alerts
- Number of Empty Values: How many empty required fields do you have as these will cause data incidents or lead to poor decision-making
- Data Time-to-Value: How much time does it take to use data to enable a decision or solve a problem or perform a task?
- Data Transformation Error Rate: Applications often transform data, and if performed incorrectly, will require immediate attention
- Data Storage or Management Costs: Is the cost of data archival or maintenance rising, and why?
Data Quality Management Tools
Your business, regardless of its size, has too much data for you to manage manually.. Software is needed to perform daily, and in many cases, real-time actions for:
- Data Profiling: is this the correct data for the task?
- Cleaning, parsing, de-deduplication, and deletion.
- Archival, backup, recovery, integration, and synchronization either on request or at a set time.
- Data versioning per application and product usage.
- Data distribution to external sources or data stores.
- Data quality tasks using Artificial Intelligence (AI) and Machine Learning (ML) rules.
- Continuous data monitoring.
Summary of Data Quality Management
Your data is your main asset after people. Without quality data, your efforts to create and manage products or services, make decisions, help customers, maintain competitiveness and relevance, and meet regulatory obligations will be difficult, if not impossible.
Consider the cost of insufficient data as seen by this graphic from Ringlead:
Data quality will fluctuate as your business changes, similar to your own body’s health as conditions alter based on what you are doing or feeling. MDM frameworks and governance will benefit from holistic enterprise data management views, leading to good data quality management practices specific to your organization. The goal is to set SMART data quality metrics that help your organization do its job well while being alerted if an issue arises.
Your customers will notice if data is insufficient or wrong or misused, and their reaction can cause irreparable damage or lead to fines such as those from GDPR. Your CDO can help create the governance framework, but data quality is the role of staff and vendors. Finally, data quality management should be a board-level discussion and concern which sets the culture of data quality as part of everyone’s role, including vendors you rely upon.